Data lies at the root of digital transformation. Hence, efficient handling of large volumes of data has become critical for enterprises. The demand for real-time analysis of data from connected systems to be delivered to stakeholders is a challenging process. The need for rapid decision-making based on data and tight competition has given rise to new data management methods.
According to Gartner, being a collaborative data management practice, DataOps can improve communication, integration, and automation of data flows between data managers and data consumers across an enterprise.
DataOps has tremendous potential to leverage AI and ML to deliver insights and analytics faster to those who need them for critical purposes. It combines users, processes, and technologies to create a reliable, quality data pipeline for any user to derive insights.
DataOps for Performance Improvement
DataOps is driven by the objective of performing as a cross-functional system of working on acquiring, storing, processing, executing, and delivering quality data to the end users. It combines the efforts of software operations development teams (DevOps) and advocates sharing tools, methodologies, and organizational structures for better management and protection of processes.
To obtain faster and more reliable insights from data, DataOps teams work on a continuous feedback loop or the DataOps lifecycle. Although influenced by DevOps, DataOps incorporates different technologies and processes given the volatile nature of the data that flows into a business. Due to the lifecycle, data teams and business stakeholders can work together in tandem to deliver more reliable data and analytics to an enterprise.
DataOps Lifecycle
- The planning phase where different teams in an enterprise partner with each other to finalize data quality and availability. For instance, product, engineering, and business teams set KPIs, SLAs, and SLIs.
- Understanding how to build data products and ML that can power data applications.
- Integrating the code and/or data product within the existing tech infrastructure and or data stack.
- Repeated data testing to ensure it matches business logic and meets operational requirements of data needed.
- Releasing this data into a test environment and slowly merging it into production.
- Running this data on applications such as Looker or Tableau dashboards and data loaders that feed machine learning models.
- Continuously monitor and observe for alerts for any anomalies in the data.
This is a repetitive cycle that uses similar principles of DevOps to data pipelines to help data teams collaborate better such that they can identify, resolve, and possibly prevent data quality issues from occurring at all.
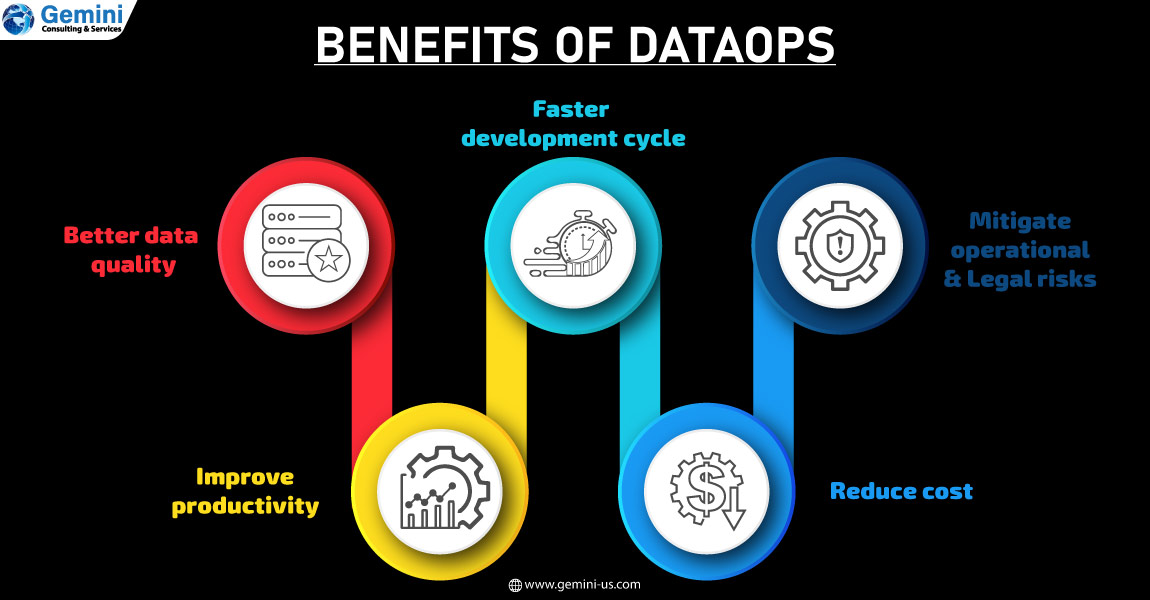
- Ensures better data quality by automating routine testing and introducing end-to-end observability with monitoring and alerting across every layer of the data stack. This reduces opportunities for human error and empowers data teams to proactively respond to data downtime incidents.
- Better productivity due to the automation of tedious engineering tasks, such as continuous, quality checks of codes and anomaly detection. Resources can focus their valuable time on improving data products, building new features, and optimizing data pipelines.
- Since multiple teams work simultaneously on projects to deliver results, development cycles are shorter, and this reduces costs. DataOps helps teams obtain faster insight, accurate analysis, improved decision-making, and higher profitability.
- Operational and legal risks are reduced as government regulations, such as General Data Protection Regulation (GDPR) and California Consumer Privacy Act (CCPA) have amended the ways in which businesses are allowed to handle data. DataOps provides more visibility and transparency on how users handle data, which tables data feeds into, and who can enjoy access to data either up or downstream.
The ultimate objective of DataOps is to make teams capable enough to manage the critical processes, which impact the business, interpret the value of each one of them to expel data silos, and centralize them. DataOps seeks to balance innovation and management control of the data pipeline.
Enterprises should make maximum use of high-value data as they can provide great insights to grow the business. Join hands with Gemini Consulting & Services to extract maximum value from a large amount of data. Contact us to know how DataOps can help you make data-driven business decisions.
DataOps Architecture in AWS, Azure & GCP
DataOps architecture combines and applies the agile and DevOps principles to data so that it becomes easy to introduce modern paradigms of software development and data sharing. The essence of a DataOps architecture is to collaborate and bring business groups together by breaking down silos that exist between data and the business using it. It offers a common ground where people with different perspectives, data needs, and use cases can work together to obtain the greatest value out of business data.
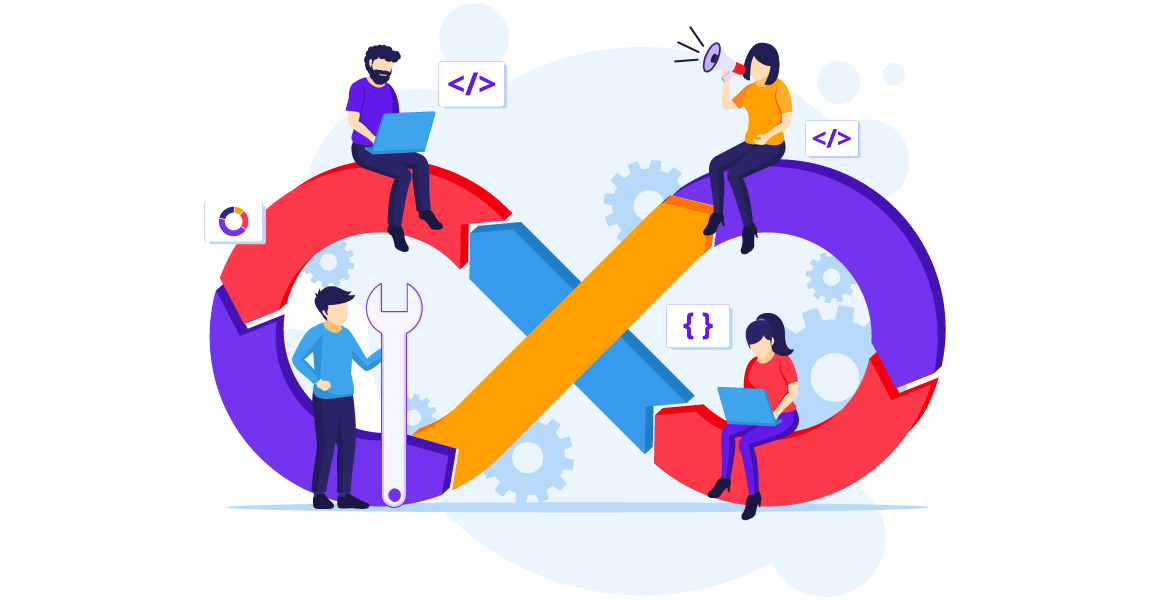
Amazon Web Services (AWS), Azure, and Google Cloud Platform (GCP) – all provide excellent tools that support the implementation of the DataOps methodology based on their cloud services. There are some set principles that DataOps follows for enhanced cloud performance such as:
- Replacing simple metadata with automated data Governance.
- Multi-dimensional agility over extensibility and scalability of data.
- Automation replaces manual data tasks.
- Multi-model data availability over a single model.
- Replacing data cleansing with a more systematic data preparation and pipeline.
DataOps focuses on business goals and data management and improved data quality. It ensures that the business goals are handled with the utmost importance, quality, and efficiency in mind. The following are some real-life use cases where DataOps have helped businesses grow and scale on AWS, Azure, and GCP.
- Improved health sector
- Making music
- Targeted marketing for diverse audiences
- Ensure that movies and TV shows are available on demand
- Greater reach among retail customers
- Improve real estate revenues
To serve the primary purpose of DataOps (accurate insights for actionable business decisions) the DataOps teams need to adhere to data governance and security principles. The data flowing across source systems, pipelines, and various workflows must have integrity and security from potential threats and leaks.