Data quality plays a pivotal role in facilitating a seamless, efficient, and expedited migration process. In its absence, businesses are unable to harness the power of analytics, potentially impeding growth for high-performance organizations.
The inherent challenge with data lies in its complexity and the potential for disorder, especially when numerous stakeholders have access to it. Additionally, the diverse array of data sources, often in various formats, further complicates matters. Moreover, data depreciates over time, making a robust application data management strategy imperative for maximizing application outputs.
According to Gartner, data quality issues can impose significant costs on organizations, estimating an average annual expenditure of $12.9 million due to poor data quality.
Given these considerations, organizations must focus on data migration between platforms with a careful assessment of increased risks, added resource demands, and potential overheads that could affect returns. Let’s delve into more specific aspects.
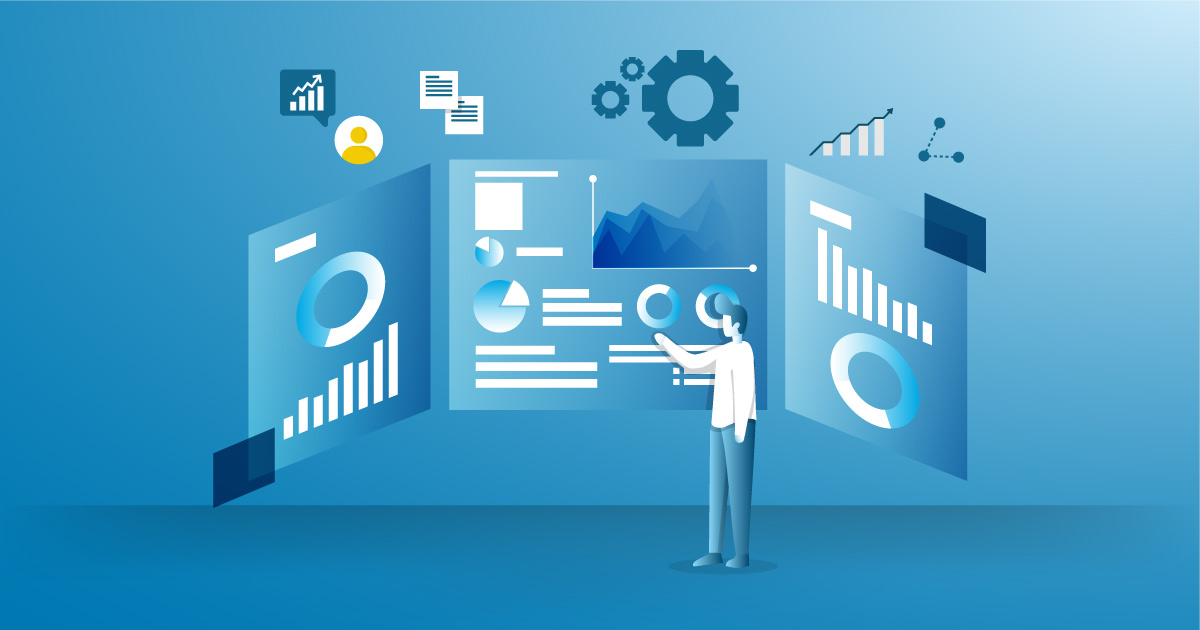
Migration to SAP Cloud
To initiate an effective data migration, organizations must first establish comprehensive control over data movement within existing applications. It’s crucial to bear in mind that the ultimate goal of migrating to S/4HANA is enhancing the performance of various business functions, including operations, reporting, compliance, and agility. Migrating data into an SAP environment begins with handling data residing in legacy applications, which may require conversion. Such data and systems often lack thorough or up-to-date documentation.
An evaluation of the current data landscape and a meticulous audit of existing data form the bedrock of a successful migration to SAP. Regardless of the chosen data migration method, maintaining data quality remains non-negotiable. Data must fulfill the following objectives:
- Minimize business risk during the transition to SAP.
- Furnish high-quality data for testing and the go-live phase.
- Collect and organize data rules in advance for post-go-live application.
Assessing Data Quality
During an organization’s SAP S/4HANA journey, project teams often concentrate on critical functional builds and testing requirements necessary for program success. A robust testing program may identify data issues before the go-live, but this may still result in timeline delays to rectify these issues before the new system’s launch. Establishing a solid data conversion strategy well before the project’s initiation is imperative. Addressing data preparation and data conversion concerns in a methodical manner before the project’s commencement greatly benefits the project team and contributes to overall program success.
To reiterate, data quality entails accuracy, completeness, consistency across platforms, timely delivery, and authenticity.
Emphasizing data as a top priority during S/4HANA implementation, alongside functional and business process considerations, is crucial. Gemini Consulting & Services has a team of experts who have successfully completed over 20 migrations to the S/4HANA environment. We can ensure that your business does not get impacted due to poor data quality during an S/4HANA migration. Contact us to get further insights on how we can ensure data quality.
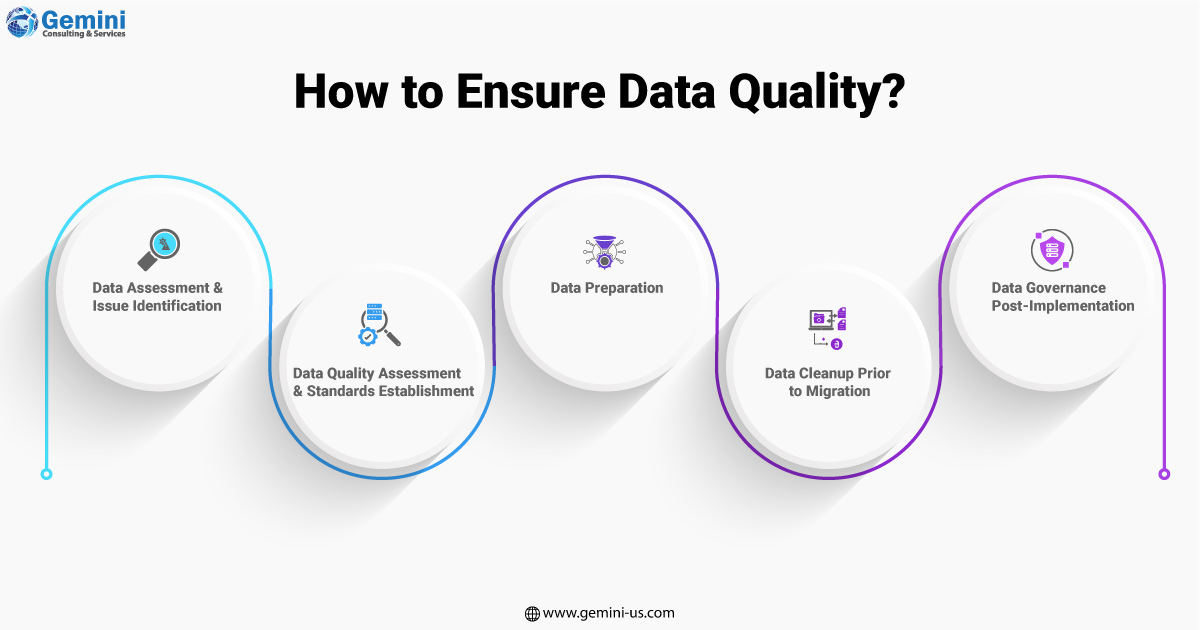
Digital transformation initiatives, such as migrating to S/4HANA, inherently involve data transformation efforts that infuse technology across all facets of a business, spanning HR, marketing, operations, and more. Given below are the key steps in this process.
- Data Assessment and Issue Identification
Begin by comprehending the flow of data in and out of your systems while evaluating ongoing data-related challenges. This initial step creates a baseline understanding of your data landscape, reducing the time and resources required during the migration. Data profiling is crucial, as it aids in identifying relevant data, ensuring that legacy data insights are leveraged effectively, and facilitating data harmonization and cleansing for necessary data only.
- Data Quality Assessment and Standards Establishment
Data projects often falter due to data discrepancies in scattered spreadsheets and systems. Validate data against existing business rules to maintain migration consistency and minimize confusion at every project phase. Establish a collaborative process between existing data and S/4HANA, ensuring that rules and mappings remain usable post-go-live. Data quality standards, like harmonization and standardization, enhance real-time insights and provide room for improvement, reducing migration timelines and risks. This phase also offers an opportunity to refine the overall enterprise data strategy, particularly in terms of clear data governance rules.
- Data Preparation
Many enterprises grapple with challenges before migrating to S/4HANA, including unclear data definitions or standards, duplicate or incomplete data, and unresolved historical business process changes affecting legacy data. Migration efforts should encompass the future vision of the business, not just its current state. Preparing data involves strategizing and cleansing it before conversion. Effective tools like SAP Data Services and SAP Information Steward can be employed for data cleansing, making use of available SAP licenses.
- Data Cleanup Prior to Migration
After establishing an efficient extraction process, focus on data profiling and cleansing while keeping sight of relevant business processes. Design business rules tailored to assess data quality, considering how master data elements (e.g., customer and material) impact critical processes like order-to-cash. Customize data quality checks to align with specific business needs. Master data plays a pivotal role in system behavior and can be configured to enforce business rules on other essential SAP master data fields. Implement a remediation plan and utilize data quality reports, such as a data readiness index (DRI), to manage this process effectively. Lastly, execute data mapping from old data structures to proposed S/4 structures, encompassing the entire migration process.
- Data Governance Post-Implementation
Maintain data quality by implementing data governance processes after going live. While commencing with clean data is commendable, it’s equally crucial to ensure efficient processes, defined data quality rules, and clear stewardship of master data to prevent future data issues.