Artificial intelligence (AI) is proving to be a major trend rather than a passing fad. Gartner suggests that by 2026, over 80% of enterprises are expected to deploy some form of generative AI APIs or applications. To join this majority, businesses need to decide on the optimal approach for training and deploying AI either on-site or in the cloud.
Training AI necessitates specialized and costly hardware, starting from mid-six figures and potentially reaching several million dollars. Unlike standard server equipment, this hardware is not versatile for other tasks such as database management. Moreover, AI training is a resource-intensive process, often taking weeks or even months depending on the dataset size. Most of businesses cannot afford to spend this kind of time.
Given these challenges, companies have two primary choices: Invest in their own AI hardware infrastructure or opt for AI-as-a-service (AIaaS) providers. While leading cloud providers offer AIaaS, the segment is increasingly witnessing several smaller vendors as well. These providers not only lease the necessary hardware but also offer prebuilt models. This leads to reduction in time for deployment. This approach can be more cost-effective and faster to implement than managing AI infrastructure independently.
AI as a Service (AIaaS) offers cloud-based access to integrate and utilize AI capabilities in projects or applications, eliminating the need for organizations to establish and manage their own AI infrastructure. It provides prebuilt and pretrained models, such as for chatbots, sparing users the effort of training their own models.
The primary advantage of AIaaS lies in avoiding the substantial costs associated with on-premises AI hardware. For instance, Nvidia's DGX Pod H100 server starts at $500,000, with larger setups like the SuperPOD beginning at $7 million. This cost can be frightfully expensive for many businesses, particularly smaller ones, making AIaaS the more viable option.
Another advantage of AIaaS is its flexibility for experimentation, enabling users to pay solely for the resources they consume. This contrasts with the significant upfront investment and extended lead times required for purchasing and maintaining on-premises AI hardware, which can be affected by supply limitations.
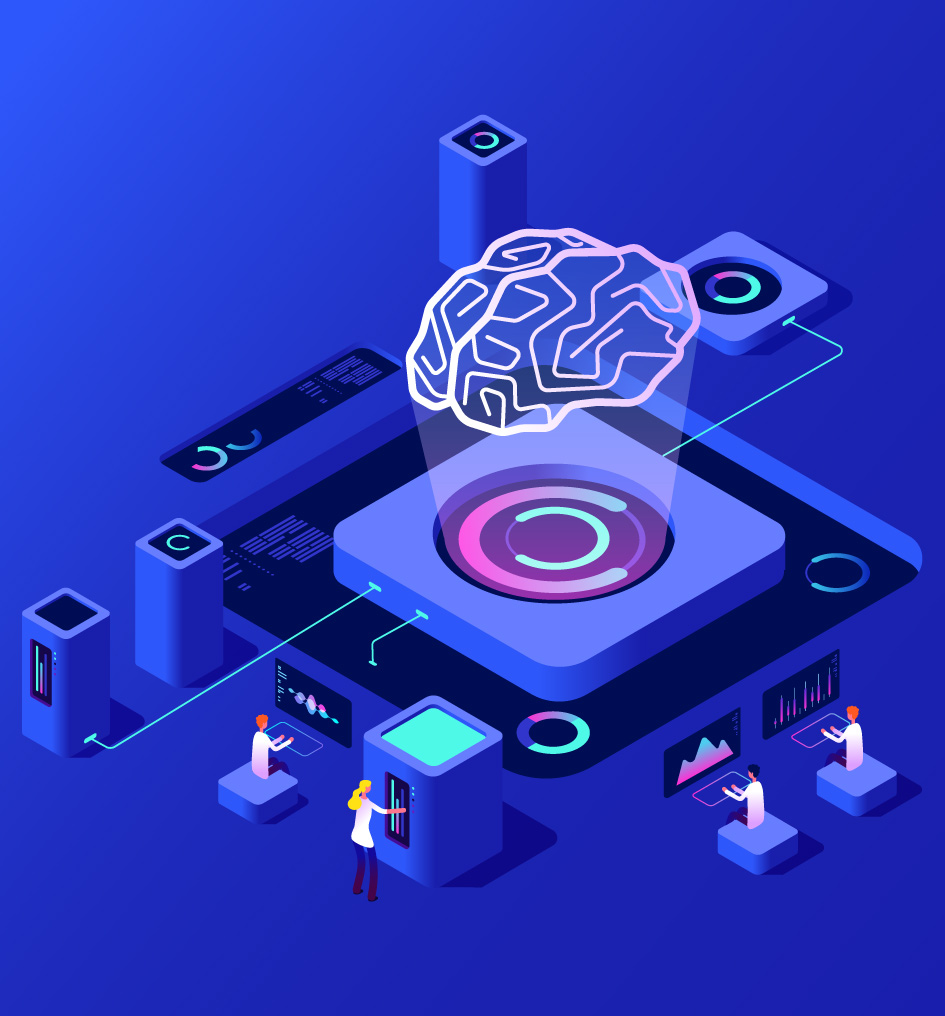
Key advantages of using AIaaS
- Lower Barrier to Entry: AI hardware is costly compared to standard servers, but using AIaaS is still more economical than purchasing dedicated hardware.
- Accelerated Time to Market: Establishing AI hardware on-site can be lengthy and demands specialized knowledge. AI-as-a-Service (AIaaS) providers manage these challenges, enabling companies to concentrate on their primary business activities.
- Access to Advanced Technology: AIaaS providers frequently refresh their services, giving users access to the latest AI innovations without requiring internal system updates.
- Scalability: AI-services hosted in the cloud enables enterprises to scale resources up or down easily based on their business requirements, offering greater flexibility and adaptability.
- Access to AI Expertise: AI talent is scarce, and AIaaS providers offer expertise in managing AI infrastructure that may not be available in-house.
Challenges of AIaaS
- Vendor Lock-in: Transitioning between AIaaS providers can be complex due to differences in platforms and APIs.
- Restricted Customization: Off-the-shelf AI models might not fully meet unique business requirements, which could necessitate custom solutions or modifications.
- Security and Privacy Concerns: Entrusting data to third-party providers raises considerations around data security and compliance.
Ultimately, leveraging AIaaS allows organizations to offload the complexities of AI infrastructure management, enabling faster innovation and deployment while managing costs effectively.
When considering AI as a Service (AIaaS), there are several crucial aspects to evaluate. Application-level integration, model engineering capabilities, and customized model development are the three options offered by AIaaS providers.. For enterprises at lower maturity levels looking to venture into AI, starting at the application layer is advisable. Alternatively, organizations can opt for deeper integration to manage their own models.
Gemini Consulting & Services has a team of AI consultants who can assist enterprises in selecting the right AIaaS provider. Contact us to implement and develop customized AI solutions for your enterprise.
Businesses typically have access to services such as preparing unstructured data and training models using either their own data or prebuilt AI models offered by AI-as-a-Service (AIaaS) providers. These prebuilt models, developed with large datasets, can perform a range of tasks including image recognition, data analysis, natural language processing, and predictive analytics.
Access to AIaaS is facilitated through APIs or user interfaces, enabling seamless integration into existing applications with minimal programming effort.
Most providers offer a pay-as-you-go model that can vary from metered usage or a fixed monthly fee. For instance, Nvidia charges a flat rate of $37,000 per month for its DGX Cloud service.
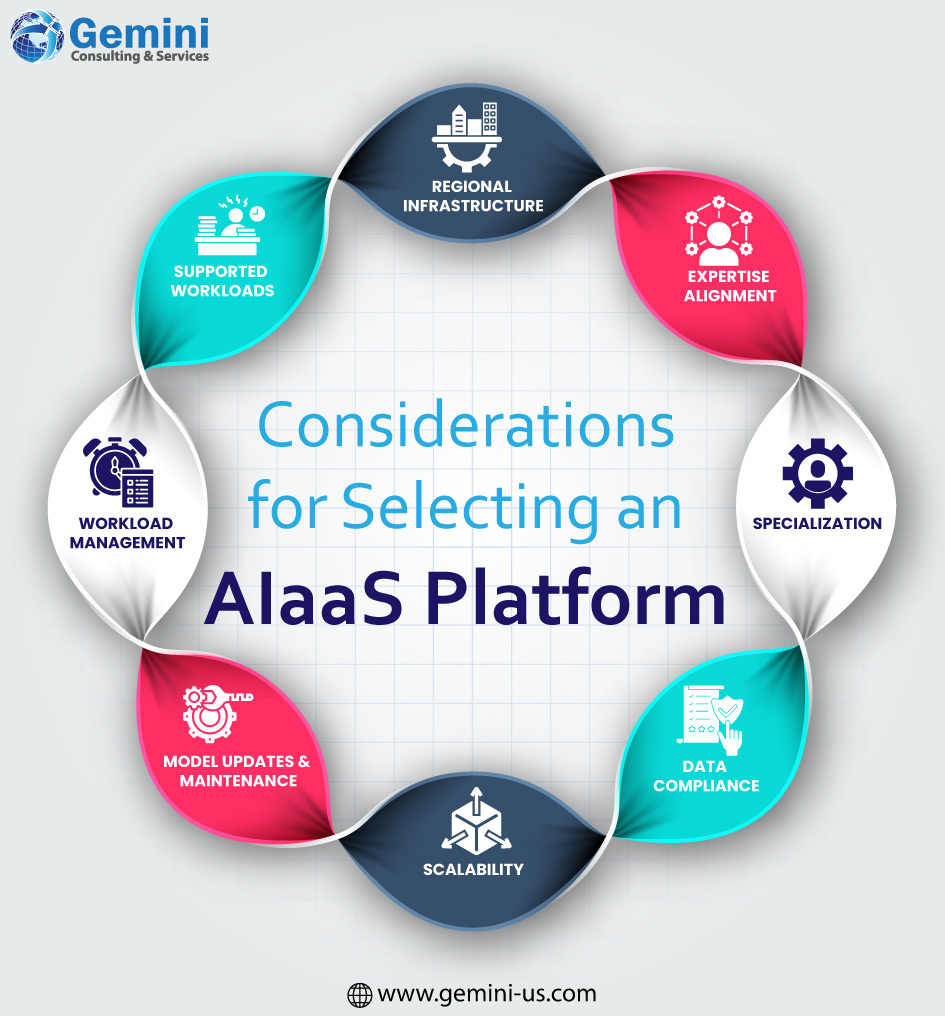
Key Considerations When Selecting an AIaaS platform
Supported Workloads: Verify that the provider covers every phase of AI deployment, including data preparation, model training, and inference.
Regional Infrastructure: Assess whether the provider's infrastructure meets your scalability needs across different global regions.
Expertise Alignment: Look for providers with experience relevant to your industry or specific challenges, supported by case studies and customer testimonials.
Specialization: You need to ensure that the provider's AI specialization is in line with the type of AI applications that you plan to develop for your company.
Data Compliance: Ensure the platform supports your data format and complies with relevant regulations, especially if dealing with highly regulated data.
Scalability: You need to look for a provider who can offer services that are scalable and grow with your enterprise requirements.
Model Updates and Maintenance: Clarify policies regarding model updates, maintenance, and the possibility of relocating models on-premises if needed.
Workload Management: Assess the provider's workload management tools, particularly their ability to handle interruptions and restart processes seamlessly.
By evaluating these criteria, enterprises can effectively choose an AIaaS provider that aligns with their specific needs and facilitates successful AI integration and deployment.